The complex issue surrounding system level diagnostics
The high-tech industry is slowly but surely moving towards a situation where increasingly complex machines and systems have to be maintained. Carmen Bratosin, Senior Research Fellow at TNO and working in the ESI program, explains during the Design Automation & Embedded Systems event more about the rise of models that make predictions at the system level.
By: Dimitri Reijerman
The expertise team at ESI focuses on various diagnostic issues, Bratosin tells FHI: “Many systems are becoming increasingly complex. We conduct our research at ESI together with our partners, including ASML, Philips, Cannon and Thales. The old way of performing maintenance and diagnostics, by examining the entire system, no longer works well. Existing AI technology in this area also often does not work well in practice. That's because you don't want to let a machine crash to collect data, which means there is insufficient data about malfunctions of a specific machine.”
ESI looks at digital twins, but also at other models to predict and prevent disruptions: “One option is to use a knowledge-based model, model-driven systems engineering,” says Bratosin. “A system is built based on a model. These models are constantly verified and refined. The question is whether you can also use these models to perform diagnostic tasks. That is what we are working on at ESI with our partners.”
“We currently have two projects underway. For example, you can generate a model from electrical diagrams that can be used for faults in the field. This is still at prototype level, but it can help a technician identify a fault. This gives us sufficient confidence that this method works. The question now is whether we can extend this methodology to system level, up to the scale of a complex ASML chip machine.”
Problems to solve
“The challenge is to capture the physical relationships in the machines but not to do that in a physical model. The machines are simply too complex for that,” says Bratosin. “So the big question is to what level you should abstract. We also examine whether these models can also be used on other types of machines. To achieve this, we have to carry out projects with other partners.”
“We see that there is a lot of international interest in predictive maintenance. You could even call it a hype. But many companies that used older AI algorithms for this have now disappeared. We also hear from our partners that they are dissatisfied with these techniques.”
Less e-waste
“With this technology we also want to help with further greening,” the researcher explains. “With many malfunctions, multiple parts are replaced, although they later turn out not to be defective. This creates a lot of e-waste. With the first time right method you can repair faster and cheaper with a minimum number of adjustments.”
During her lecture, Bratosin will want to make it clear that solutions are in sight: “Ultimately, I want to make it clear during my presentation that it is becoming increasingly difficult to maintain complex systems. But thanks to developments in the field of deep learning and graph reasoning, solutions are in sight. We are working on this together with our partners.”
Would you this lecture to attend? Register for free for a visit to the D&E event 2021.
Related companies
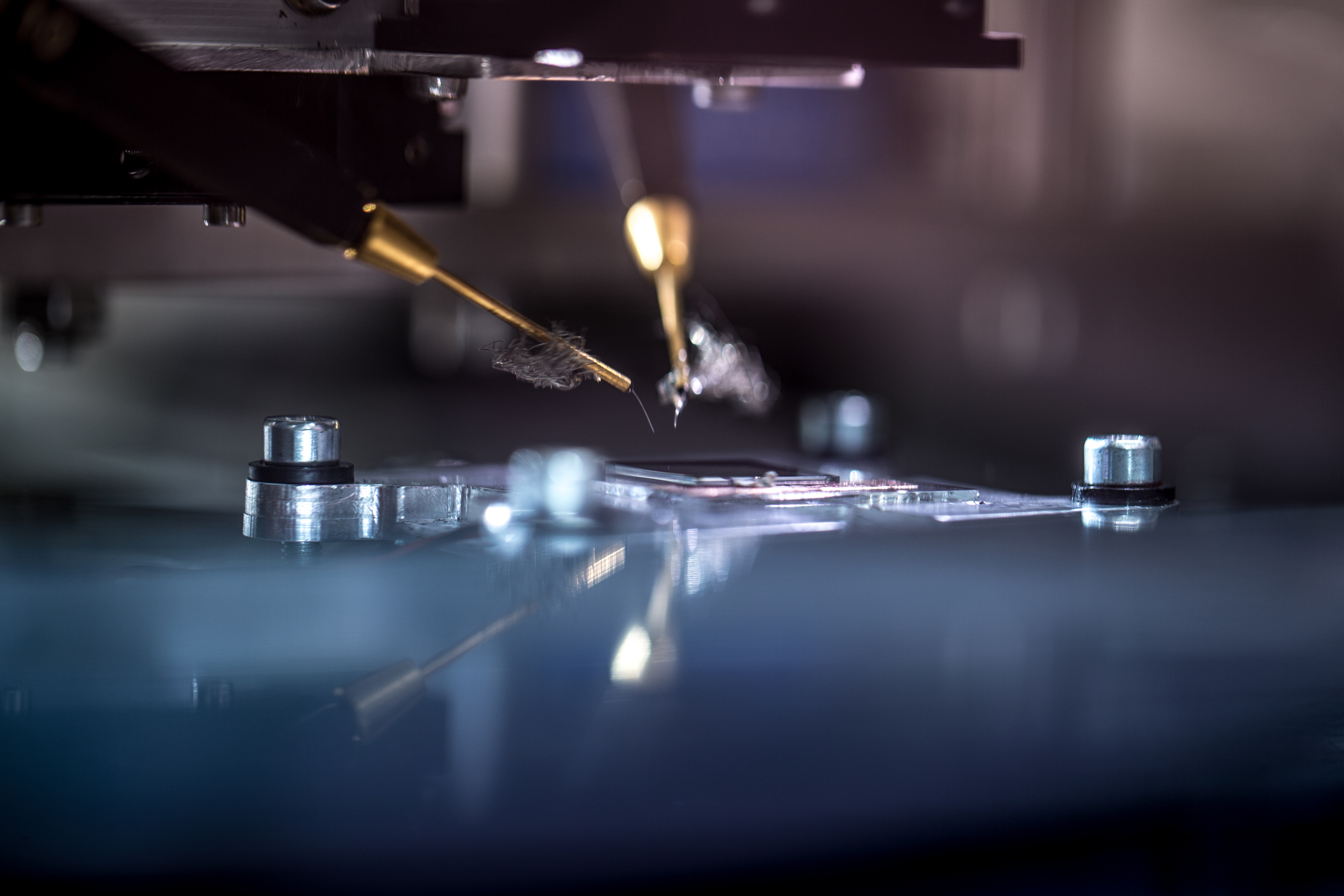
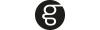