The extensive automation of laboratories is now widely used. Artificial intelligence (AI) is playing an increasingly important role in this. And developments are happening at breakneck speed.
By: Dimitri Reijerman
In healthcare and pharmaceuticals, spectacular results are being achieved by using the predictive value of well-trained AI models in particular. A good example is research conducted by scientists from the Mahmood Lab at Brigham and Women's Hospital. They developed an AI model based on data from the Cancer Genome Atlas Program, genomic sequencing, pathology and patient history. Based on information from multiple sources, the algorithm can predict the course of fourteen different types of cancer better than human specialists.
Artificial intelligence is also strong and already well developed in tasks related to image recognition. In the United Kingdom, an algorithm was tested to determine whether someone has (early) dementia and at what rate the disease will manifest itself based on just a brain scan. Other models have predictive value. The British DeepMind developed a model that was able to predict the composition of proteins thanks to AI, followed by the AlphaFold algorithm that indexed 350,000 protein structures. This data helps to better understand diseases and develop medicines faster, but also enables scientists to understand the immune system of the honeybee, for example.
It is clear that artificial intelligence is indispensable for scientific progress. But investments in AI are also essential for companies that want to remain relevant and profitable. In 2021, CBS found that almost half of all large companies used one or more Artificial Intelligence technologies. That number has probably only increased.
In the lab
In the laboratory world, traditionally a fairly conservative market, more and more parties are also opting for investments in and the use of artificial intelligence. Take the AI4B.io Lab on the Biotech Campus Delft. This project was announced in January 2022 – where AI4B.io stands for Artificial Intelligence Laboratory for Biosciences – with millions of investments by DSM and TU Delft. According to the parties, this laboratory is the first of its kind in Europe. The aim is to apply AI to bioproduction, such as the development of microbial strains and large-scale industrial process optimization. By integrating life sciences and digital technologies, the parties hope to shorten innovation cycles. Long-term lab experiments could well become a thing of the past.
Money is also available from the government. For example, the National Growth Fund has invested 97 million euros in Robotlab, which describes itself as 'the revolution of self-thinking molecular systems'. In this research project, which has been set aside for seven years, an attempt is being made to develop a fully automated laboratory. Among others, Fontys University of Applied Sciences Eindhoven and Radboud University are closely involved.
Interesting in this project is the combination of high-tech (robotics) and artificial intelligence to design complex molecular mixtures. The researchers want to use AI to conduct more efficient research into new chemical products based on analyses and big data instead of trial and error and human intuition. Moreover, this should result in more sustainable products such as paints, glues, drinks, vaccines and cosmetics.
Reverse side
Although the rise of artificial intelligence also ensures an even higher speed of innovation in lab automation, there are also dangers lurking. The field is mainly warning about the big tech companies. For example, the aforementioned DeepMind is a subsidiary of Alphabet, the parent company of Google. Due to the enormous computing power of the supercomputers, a lead in the development of AI, deep pockets and substantial investments by the American tech giants, scientists fear that there will be a great dependency on 'big tech'. However, there are often no direct alternatives yet.
Another challenge is structuring data. Algorithms are often trained on the basis of enormous datasets. In order to make these bundles of data interchangeable for other and future research, standardization is essential. One of the possible solutions for this is the application of the so-called FAIR principle, in which guidelines have been drawn up for the way in which data is described, stored and published.
In conclusion, it can be stated that AI-driven innovation has a huge impact in many sectors – apart from the social impact – and will only continue to grow. In the lab world, where robotization has already achieved significant efficiency gains, artificial intelligence can be used to conduct (scientific) research even faster and smarter. The role of the researcher is also changing, says TNO. And sometimes in unexpected ways. It remains important for the Netherlands and the EU to continue to invest heavily in these technologies in the coming years. Because missing the boat here can result in a backlog that can no longer be made up.
Related companies
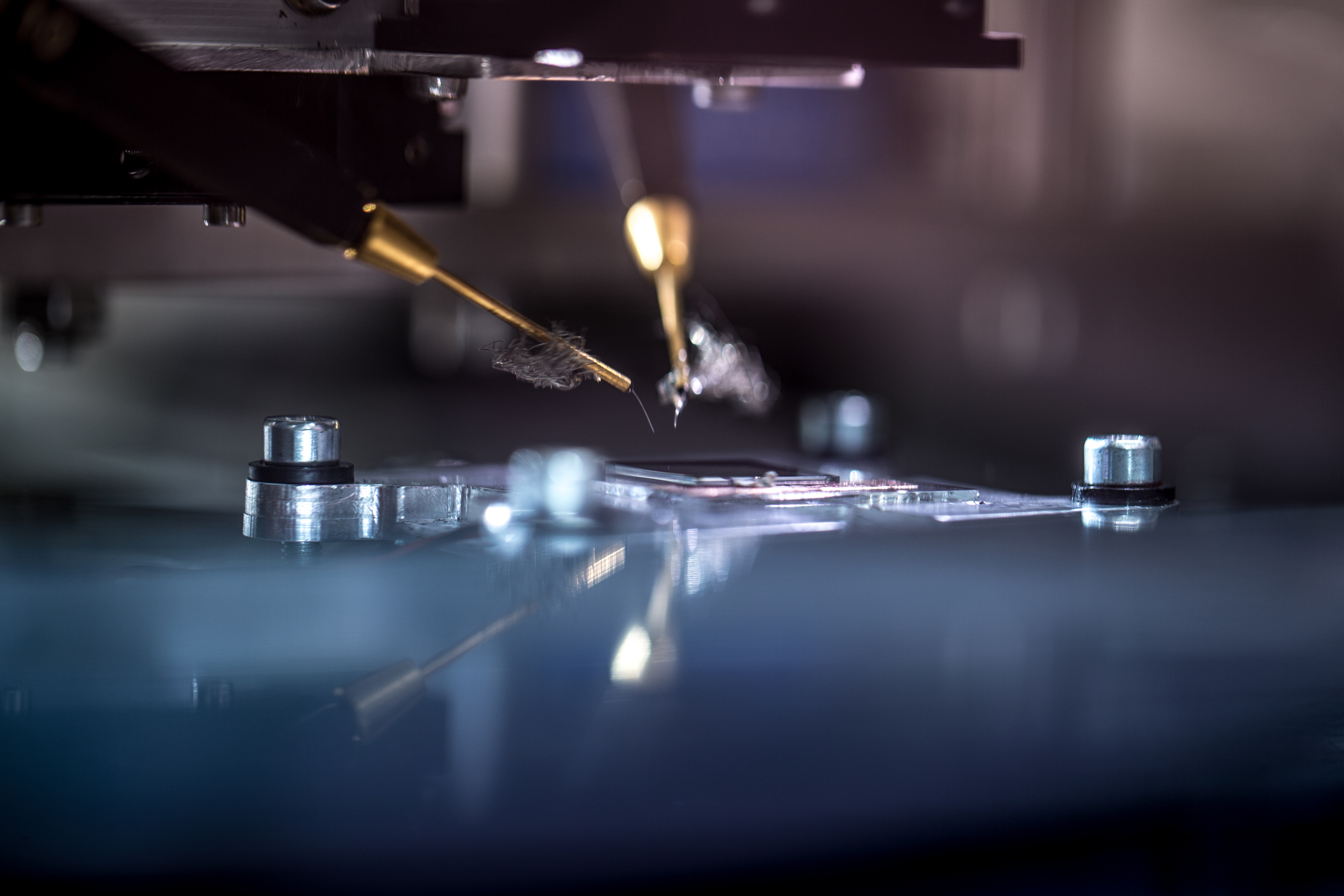
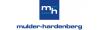